Intro
Discover 5 ways to compare columns efficiently, leveraging data analysis, spreadsheet tools, and table comparisons to reveal insights and trends, enhancing data comparison and column matching techniques.
Comparing columns is a fundamental aspect of data analysis, allowing us to identify patterns, trends, and discrepancies within datasets. Whether you're working with spreadsheets, databases, or data visualization tools, the ability to compare columns efficiently is crucial for making informed decisions. In this article, we'll delve into five ways to compare columns, exploring the benefits, limitations, and best practices for each method.
The importance of comparing columns cannot be overstated. By analyzing the differences and similarities between columns, we can gain valuable insights into our data, from identifying correlations and outliers to uncovering hidden patterns and trends. Moreover, comparing columns enables us to validate data quality, ensure consistency, and make data-driven decisions. With the increasing complexity of modern datasets, the need for effective column comparison techniques has never been more pressing.
As we navigate the world of data analysis, it's essential to recognize the various challenges associated with comparing columns. From dealing with missing or duplicate values to handling large datasets and ensuring data integrity, the process can be daunting. However, by leveraging the right techniques and tools, we can overcome these obstacles and unlock the full potential of our data. In the following sections, we'll explore five ways to compare columns, providing a comprehensive guide for data analysts, scientists, and enthusiasts alike.
Method 1: Visual Comparison
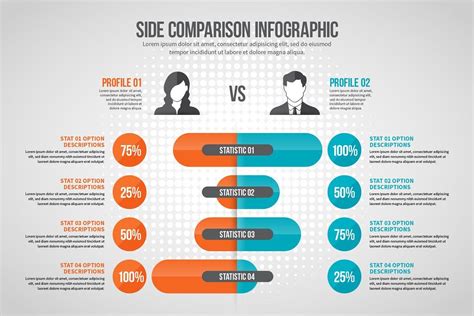
Benefits of Visual Comparison
The benefits of visual comparison are numerous, including: * Rapid identification of patterns and trends * Easy detection of outliers and anomalies * Improved understanding of correlations and relationships between columns * Enhanced data exploration and discoveryLimitations of Visual Comparison
While visual comparison is a powerful technique, it's not without its limitations: * Can be subjective, relying on individual interpretation * May not be effective for large or complex datasets * Requires specialized tools and softwareMethod 2: Statistical Comparison
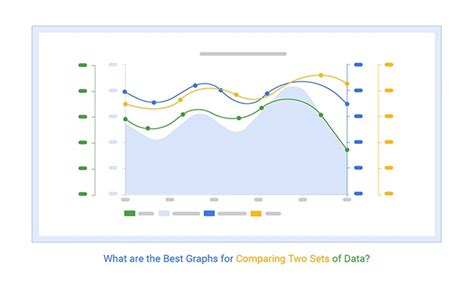
Benefits of Statistical Comparison
The benefits of statistical comparison include: * Objective and quantitative analysis * Ability to account for variability and uncertainty * Enhanced confidence in findings and decisions * Improved understanding of relationships between columnsLimitations of Statistical Comparison
While statistical comparison is a robust technique, it's not without its limitations: * Requires specialized knowledge and expertise * Can be time-consuming and computationally intensive * May not be suitable for small or incomplete datasetsMethod 3: Data Mining Comparison

Benefits of Data Mining Comparison
The benefits of data mining comparison include: * Automated discovery of patterns and relationships * Ability to handle large and complex datasets * Improved understanding of hidden interactions and correlations * Enhanced predictive capabilitiesLimitations of Data Mining Comparison
While data mining comparison is a powerful technique, it's not without its limitations: * Requires specialized software and expertise * Can be computationally intensive and time-consuming * May produce false positives or false negativesMethod 4: Machine Learning Comparison
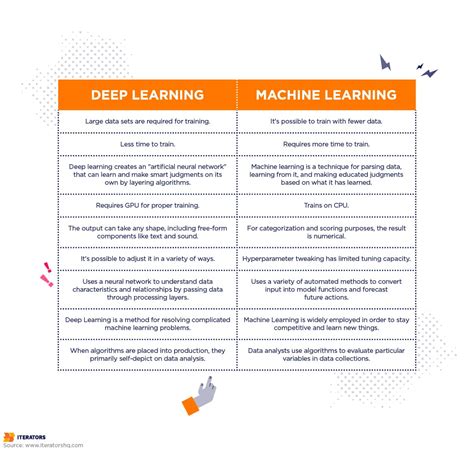
Benefits of Machine Learning Comparison
The benefits of machine learning comparison include: * Improved accuracy and robustness * Ability to handle non-linear relationships and complex interactions * Enhanced adaptability to changing data distributions * Automated prediction and classificationLimitations of Machine Learning Comparison
While machine learning comparison is a powerful technique, it's not without its limitations: * Requires large and high-quality datasets * Can be computationally intensive and time-consuming * May require specialized expertise and softwareMethod 5: Manual Comparison
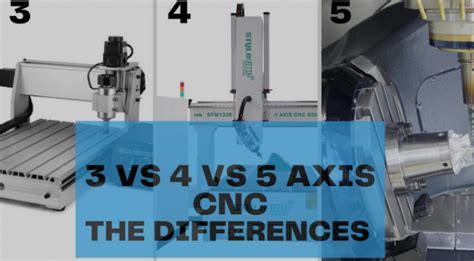
Benefits of Manual Comparison
The benefits of manual comparison include: * High degree of accuracy and control * Ability to ensure data quality and identify errors * Improved understanding of data and relationships * Low computational requirementsLimitations of Manual Comparison
While manual comparison is a simple and effective technique, it's not without its limitations: * Can be time-consuming and labor-intensive * May not be suitable for large or complex datasets * Requires manual effort and attention to detailColumn Comparison Image Gallery
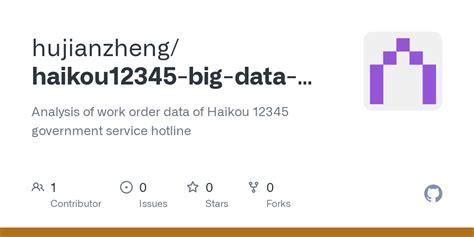
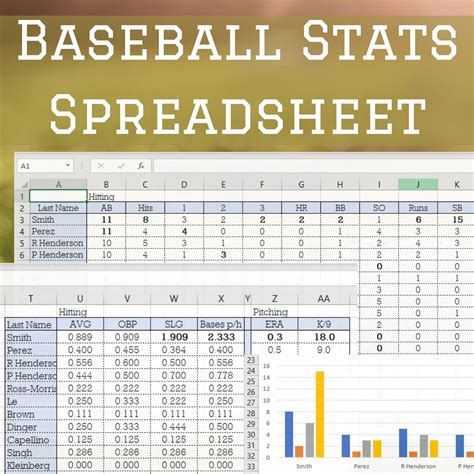
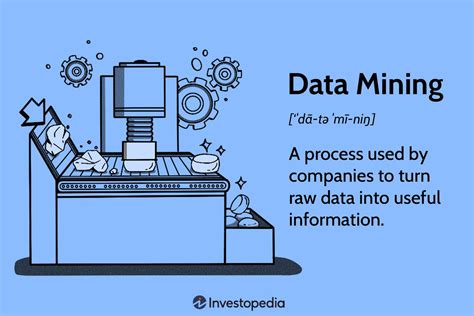
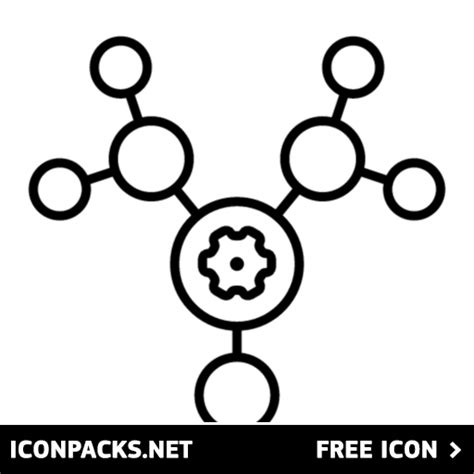
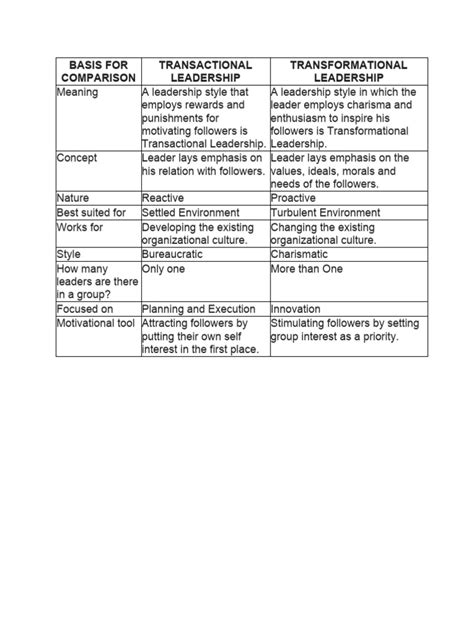
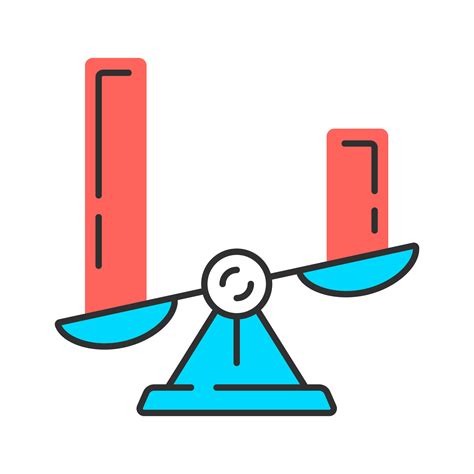
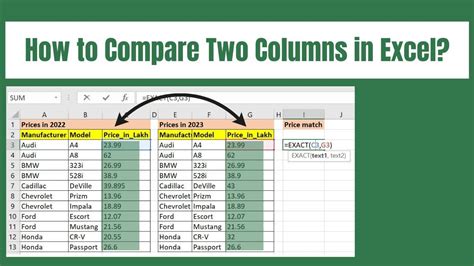
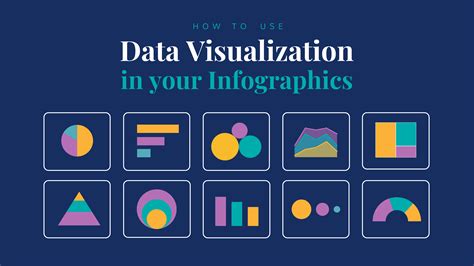
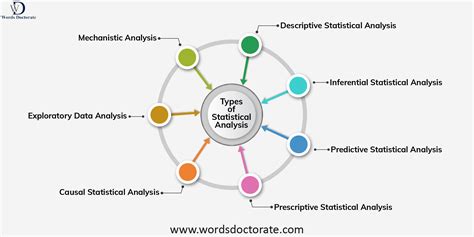
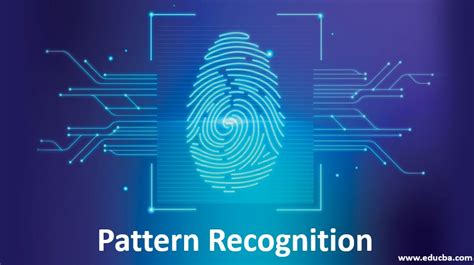
What is the best method for comparing columns?
+The best method for comparing columns depends on the specific use case and requirements. Visual comparison is suitable for exploratory data analysis, while statistical comparison provides a more rigorous and objective framework. Data mining and machine learning comparison can be used for automated discovery and prediction, and manual comparison is useful for small datasets or high-accuracy requirements.
How do I choose the right comparison method?
+Choose the right comparison method by considering the size and complexity of your dataset, the level of accuracy required, and the specific goals and objectives of your analysis. Additionally, consider the computational resources and expertise available, as well as the need for automation and scalability.
What are the common challenges in comparing columns?
+Common challenges in comparing columns include dealing with missing or duplicate values, handling large and complex datasets, ensuring data integrity and quality, and selecting the right comparison method and tools. Additionally, challenges may arise from the need for specialized expertise, computational resources, and software.
In conclusion, comparing columns is a vital aspect of data analysis, and the right approach depends on the specific use case and requirements. By understanding the benefits and limitations of each comparison method, we can choose the most suitable technique for our needs and unlock the full potential of our data. Whether you're a data analyst, scientist, or enthusiast, we invite you to share your thoughts and experiences on comparing columns, and to explore the various methods and techniques presented in this article.